Table Of Content
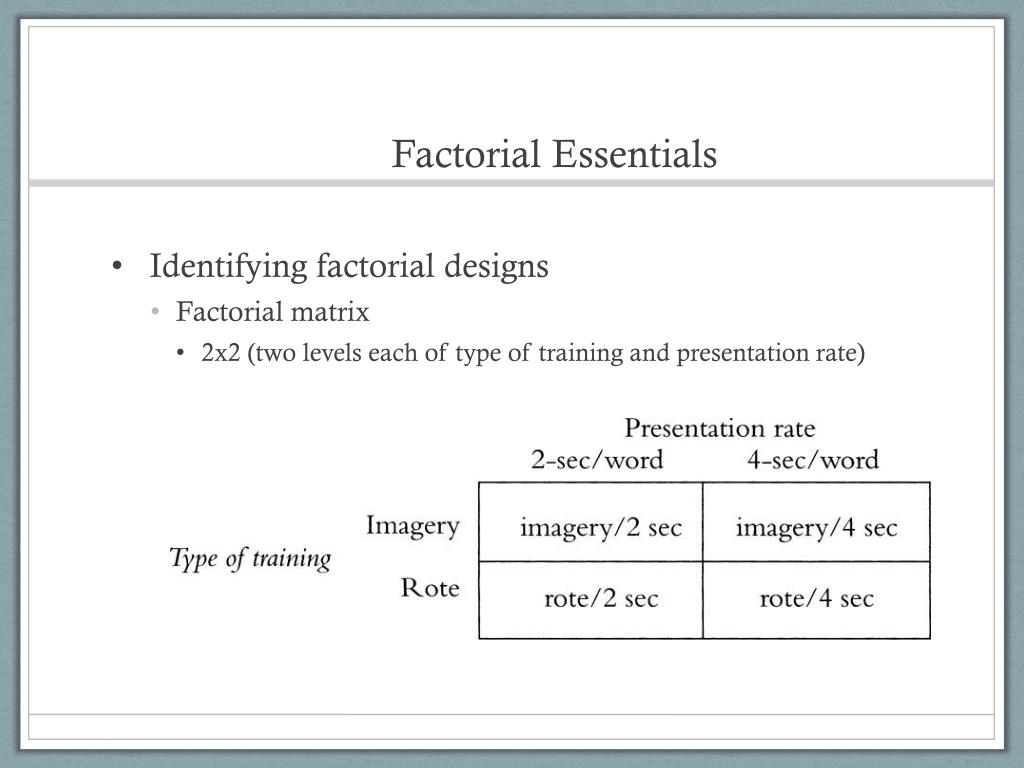
Manipulation checks become especially important when the manipulation of the independent variable turns out to have no effect on the dependent variable. Imagine, for example, that you exposed participants to happy or sad movie music—intending to put them in happy or sad moods—but you found that this had no effect on the number of happy or sad childhood events they recalled. This could be because being in a happy or sad mood has no effect on memories for childhood events. But it could also be that the music was ineffective at putting participants in happy or sad moods. A manipulation check, in this case, a measure of participants’ moods, would help resolve this uncertainty. If it showed that you had successfully manipulated participants’ moods, then it would appear that there is indeed no effect of mood on memory for childhood events.
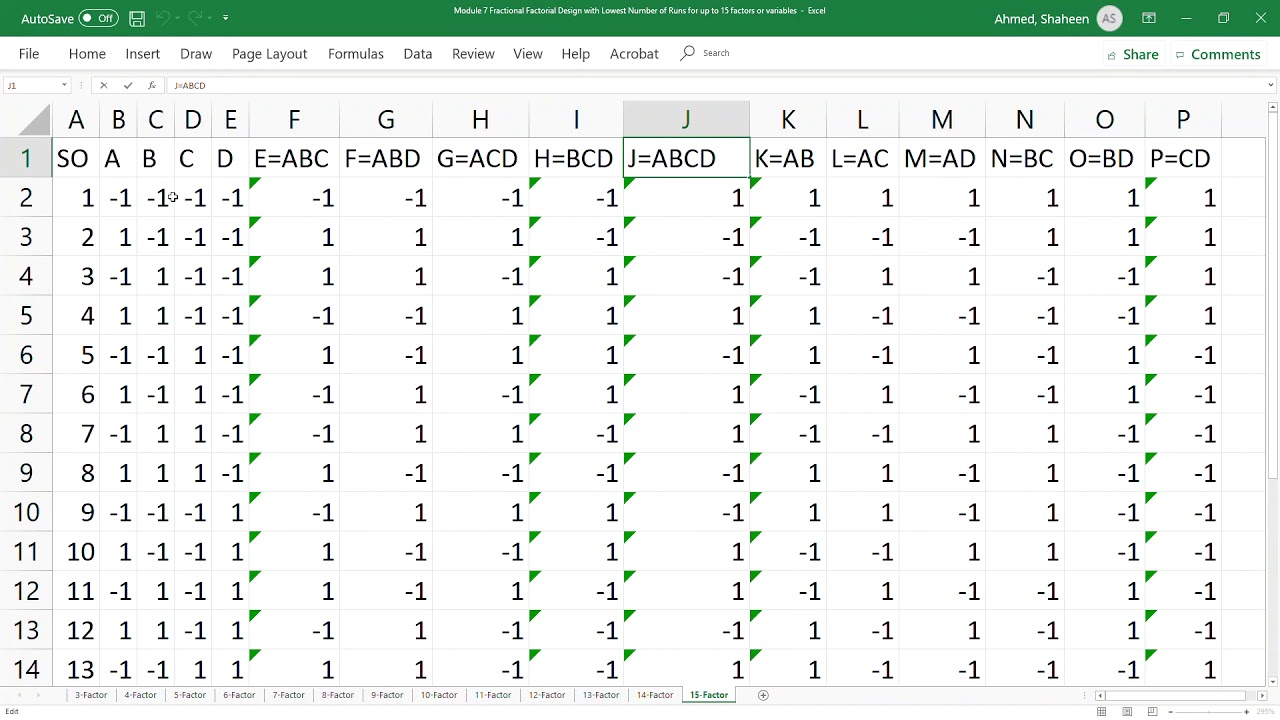
Lesson 5: Introduction to Factorial Designs
Regardless, factorial design is a useful method to design experiments in both laboratory and industrial settings. A factorial design is a type of experiment that involves manipulating two or more variables. While simple psychology experiments look at how one independent variable affects one dependent variable, researchers often want to know more about the effects of multiple independent variables.
Full factorial design and dynamic modelling of silent and ultrasound-assisted lead and cadmium removal by porous ... - Nature.com
Full factorial design and dynamic modelling of silent and ultrasound-assisted lead and cadmium removal by porous ....
Posted: Thu, 28 Apr 2022 07:00:00 GMT [source]
Main Effects
The response is only measured at four of the possible eight corner points of the factorial portion of the design. However, with this design, the main effects will be confounded with the 2-way interactions. The red bars show the conditions where people wear hats, and the green bars show the conditions where people do not wear hats. For both levels of the wearing shoes variable, the red bars are higher than the green bars. More specifically, in both cases, wearing a hat adds exactly 6 inches to the height, no more no less.
Factorial Design Example
This problem is avoided if an analysis of variance package is used, because such packages typically default to effect coding. In dummy coding, a binary variable, a reference group (e.g., a control group) is assigned a value of zero (0) and the other group (e.g., an active treatment group) is assigned a value of one (1). Effect coding of a binary variable is the same except that the zero for the reference group is replaced with −1.
Hungarian and Indonesian rice husk as bioadsorbents for binary biosorption of cationic dyes from aqueous solutions: A ... - ScienceDirect.com
Hungarian and Indonesian rice husk as bioadsorbents for binary biosorption of cationic dyes from aqueous solutions: A ....
Posted: Fri, 09 Jun 2023 19:37:38 GMT [source]
The advantages and disadvantages of these two approaches are the same as those discussed in Chapter 5. Just as including multiple dependent variables in the same experiment allows one to answer more research questions, so too does including multiple independent variables in the same experiment. For example, instead of conducting one study on the effect of disgust on moral judgment and another on the effect of private body consciousness on moral judgment, Schnall and colleagues were able to conduct one study that addressed both variables.
Look first at the effect of time since last meal only for the red bars in the “not tired” condition. The red bar in the 1 hour condition is 1 unit smaller than the red bar in the 5 hour condition. Next, look at the effect of time since last meal only for the green bars in the “tired” condition. The green bar in the 1 hour condition is 3 units smaller than the green bar in the 5 hour condition. Again, the size of the effect of time since last meal depends on the levels of the tired variable.No matter which way you look at the interaction, we get the same numbers for the size of the interaction effect, which is 2 units (i.e., the difference between 3 and 1). The interaction suggests that something special happens when people are tired and haven’t eaten in 5 hours.
When 40 year olds, however, are given a 5 mg pill or a 10 mg pill, 15% suffer from seizures at both of these dosages. There is an increasing chance of suffering from a seizure at higher doses for 20 year olds, but no difference in suffering from seizures for 40 year olds. Thus, there must be an interaction effect between the dosage of CureAll, and the age of the patient taking the drug. When you have an interaction effect it is impossible to describe your results accurately without mentioning both factors. You can always spot an interaction in the graphs because when there are lines that are not parallel an interaction is present.
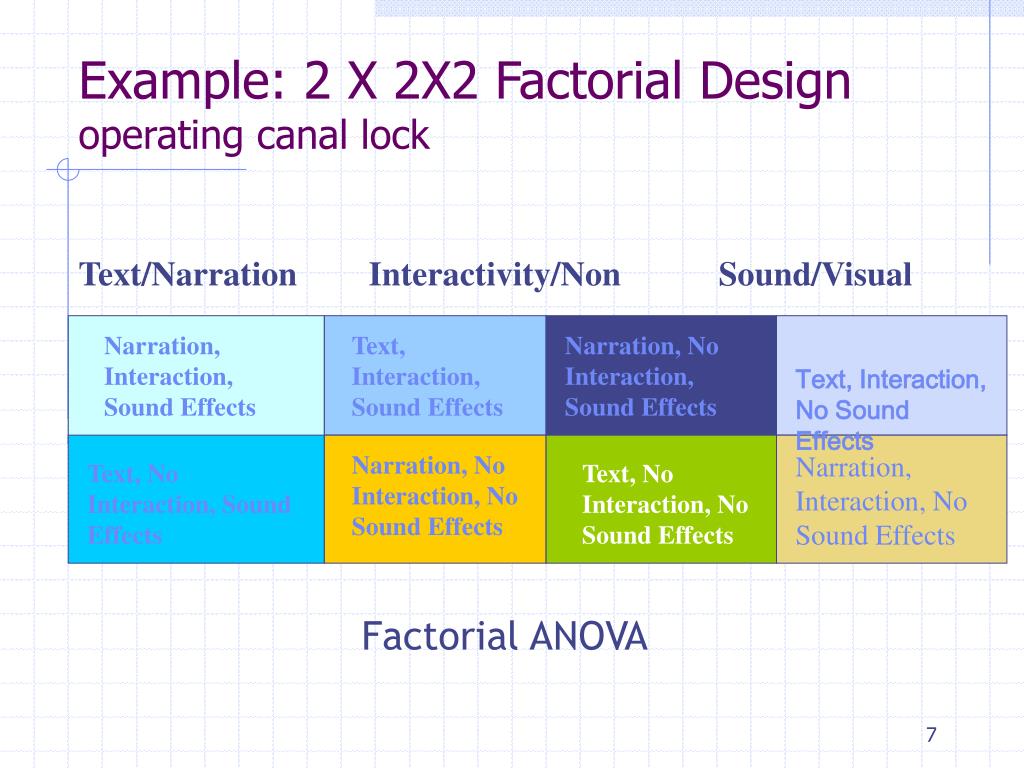
In a different but related study, Schnall and her colleagues investigated whether feeling physically disgusted causes people to make harsher moral judgments [SHCJ08]. In this experiment, they manipulated participants’ feelings of disgust by testing them in either a clean room or a messy room that contained dirty dishes, an overflowing wastebasket, and a chewed-up pen. They also used a self-report questionnaire to measure the amount of attention that people pay to their own bodily sensations. They also measured some other dependent variables, including participants’ willingness to eat at a new restaurant.
3. Factorial designs: Round 2¶
When the factors are continuous, two-level factorial designs assume that the effects are linear. If a quadratic effect is expected for a factor, a more complicated experiment should be used, such as a central composite design. Optimization of factors that could have quadratic effects is the primary goal of response surface methodology. If these values represent "low" and "high" settings of a treatment, then it is natural to have 1 represent "high", whether using 0 and 1 or −1 and 1. The full factorial design contains twice as many design points as the ½ fraction design.
Experiments that include more than one independent variable in which each level of one independent variable is combined with each level of the others to produce all possible combinations. For example, imagine that a researcher wants to do an experiment looking at whether sleep deprivation hurts reaction times during a driving test. If she were only to perform the experiment using these variables–the sleep deprivation being the independent variable and the performance on the driving test being the dependent variable–it would be an example of a simple experiment. As these researchers expected, participants who were lower in SES tended to give away more of their points than participants who were higher in SES. This is consistent with the idea that being lower in SES causes people to be more generous. But there are also plausible third variables that could explain this relationship.
So while the largest main total effect value in one set of experiments may have a value of 128, another experiment may have its largest main total effect value be 43. Frank Yates created an algorithm to easily find the total factorial effects in a 2n factorial that is easily programmable in Excel. While this algorithm is fairly straightforward, it is also quite tedious and is limited to 2n factorial designs. Thus, modern technology has allowed for this analysis to be done using statistical software programs through regression.
No comments:
Post a Comment