Table Of Content
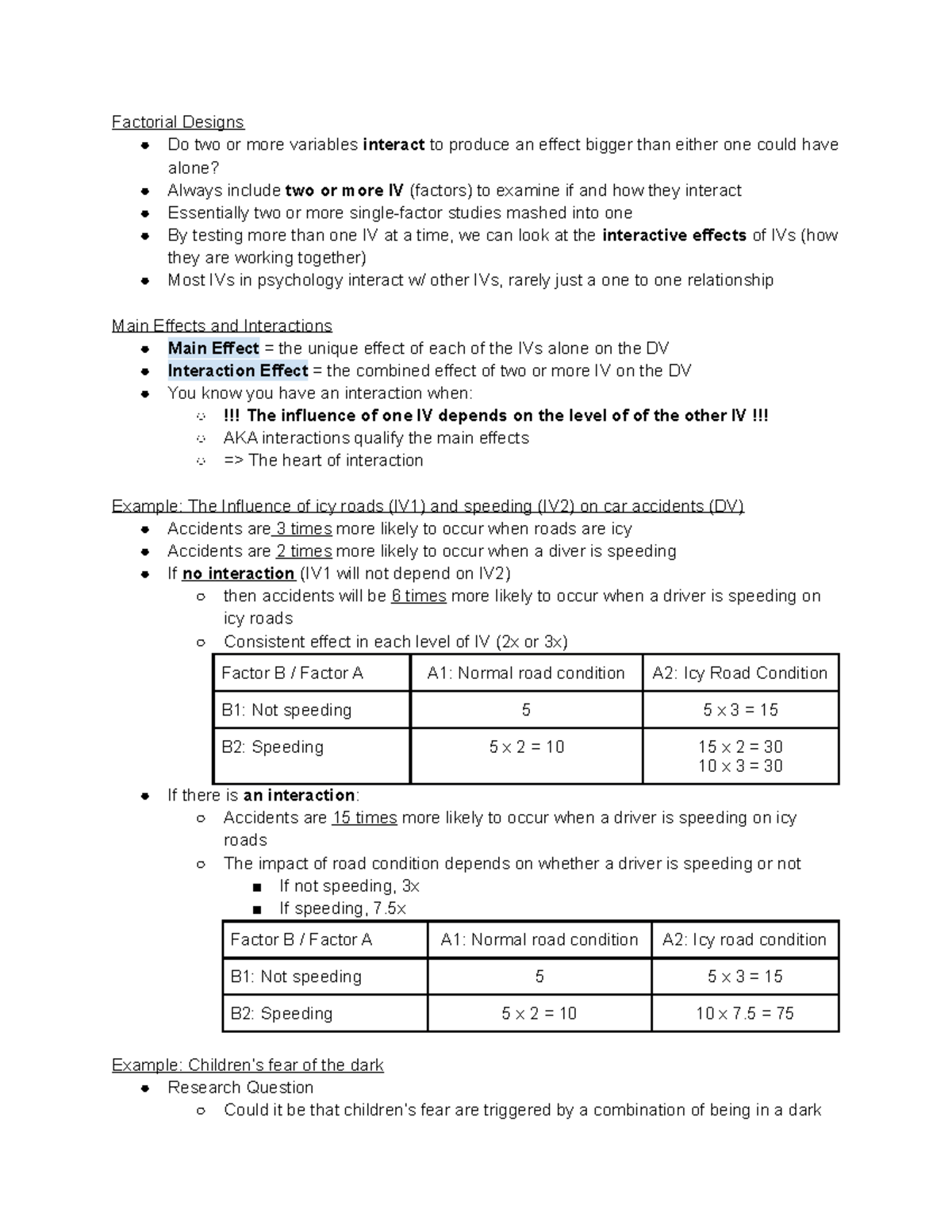
Only factorial designs permit efficient screening of multiple ICs or dimensions (e.g., length of treatment), revealing their main effects and interactions and permitting the identification of those that are compatible or most promising (Collins et al., 2016). However, factorial experiments do not permit strong inferences about how well a particular grouping of components (occurring as levels of different factors) will work as an integrated treatment as compared to a control. After all, only a small portion of a sample in a factorial experiment will get a particular set of components (e.g., in the design depicted in Table 1 only 1/32 of the N will get a particular combination of components). Recall that in a between-subjects single factor design, each participant is tested in only one condition. First, non-manipulated independent variables are usually participant background variables (self-esteem, gender, and so on), and as such, they are by definition between-subjects factors. For example, people are either low in self-esteem or high in self-esteem; they cannot be tested in both of these conditions.
Factorial experimental design for the optimization of catalytic degradation of malachite green dye in aqueous solution ... - ScienceDirect.com
Factorial experimental design for the optimization of catalytic degradation of malachite green dye in aqueous solution ....
Posted: Mon, 04 Dec 2017 12:08:50 GMT [source]
Factorial Design Example
For instance, if the purity, yield, and residual amount of catalyst was measured in the DOE study, the values of these for each trial would be entered in the columns. For example, suppose you could not conduct your design with all five factors set at their high level. The principal fraction contains this point, but the third fraction does not. You can either run the full factorial design or a fraction of the factorial design.
3.10. Interpreting main effects and interactions¶
For example, an experiment could include the type of psychotherapy (cognitive vs. behavioral), the length of the psychotherapy (2 weeks vs. 2 months), and the sex of the psychotherapist (female vs. male). This would be a 2 x 2 x 2 factorial design and would have eight conditions. In practice, it is unusual for there to be more than three independent variables with more than two or three levels each. In Chapter 1 we briefly described a study conducted by Simone Schnall and her colleagues, in which they found that washing one’s hands leads people to view moral transgressions as less wrong [SBH08].
Factorial Design Example Revisited
Instead, it involves measuring several variables, often both categorical and quantitative, and then assessing the statistical relationships among them. These included health, knowledge of heart attack risk factors, and beliefs about their own risk of having a heart attack. They found that more optimistic participants were healthier (e.g., they exercised more and had lower blood pressure), knew about heart attack risk factors, and correctly believed their own risk to be lower than that of their peers. As with simple designs with only one independent variable, factorial designs have the same basic empirical question. Did manipulation of the independent variables cause changes in the dependent variables?
But in some other imaginary universe, it could mean, for example, that wearing a shoe adds 1 to your height when you do not wear a hat, but adds more than 1 inch (or less than 1 inch) when you do wear a hat. This thought experiment will be our entry point into discussing interactions. A take-home message before we begin is that some independent variables (like shoes and hats) do not interact; however, there are many other independent variables that do. As a further example, the effects of three input variables can be evaluated in eight experimental conditions shown as the corners of a cube. Shows how each level of one independent variable is combined with each level of the others to produce all possible combinations in a factorial design. A factorial design is type of designed experiment that lets you study of the effects that several factors can have on a response.
5.1. Correlational Studies With Factorial Designs¶

The difference between red and green bars is small for level 1 of IV1, but large for level 2. The differences between the differences are different, so there is an interaction. For example, both the red and green bars for IV1 level 1 are higher than IV1 Level 2. And, both of the red bars (IV2 level 1) are higher than the green bars (IV2 level 2). The second way of looking at the interaction is to start by looking at the other variable. For example, does the effect of time since last meal depend on the levels of the tired variable?
Examples of Factorial Designs
Instead, factors are constructs that operate independently of each other. So people who are high in extraversion might be high or low in conscientiousness, and people who like reflective and complex music might or might not also like intense and rebellious music. The second point is that factor analysis reveals only the underlying structure of the variables. It is up to researchers to interpret and label the factors and to explain the origin of that particular factor structure. For example, one reason that extraversion and the other Big Five operate as separate factors is that they appear to be controlled by different genes [PDMM08]. When researchers combine dependent variables in this way, they are treating them collectively as a multiple-response measure of a single construct.
For this reason, the researcher might have participants complete the paper-and-pencil Perceived Stress Scale and also measure their levels of the stress hormone cortisol. If the researcher finds that the different measures are affected by exercise in the same way, then he or she can be confident in the conclusion that exercise affects the more general construct of stress. Finally, it is important to note that if investigators include multiple, discrete IC’s in a factorial experiment the effects of the individual ICs may be limited to the extent that the various ICs exert their effects via similar or redundant pathways (Baker et al., 2016). Thus, to the extent that two ICs affect coping execution or withdrawal severity, their co-occurrence in the experiment could reduce estimates of their main effects via negative interaction. One might think of this as interventions “competing” for a limit subset of participants who are actually capable of change or improvement; in a sense this subsample would be spread across multiple active intervention components.
If we were trying to understand how paying attention works, we would then need to explain how it is that reward levels could causally change how people pay attention (because science is cumulative). We would have some evidence that reward does cause change in paying attention, and we would have to come up with some explanations, and then run more experiments to test whether those explanations hold water. In a 2-level full factorial design, each experimental factor has only two levels. The experimental runs include all combinations of these factor levels.
The first run (as specified by the random run order) should be performed at the low levels of A and C and the high levels of B and D. In the main "Create Factorial Design" menu, click "OK" once all specifications are complete. The following table is obtained for a 2-level, 4 factor, full factorial design. None of the levels were specified as they appear as -1 and 1 for low and high levels, respectively. The following Yates algorithm table was constructed using the data from the interaction effects section. Since the main total factorial effect for AB is non-zero, there are interaction effects.
When you look for the differences, it feels like you are doing something we would call “paying attention”. If you pay attention to the clock tower, you will see that the hands on the clock are different. We could give people 30 seconds to find as many differences as they can. So, our measure of performance, our dependent variable, could be the mean number of differences spotted.
By adding up the coefficient effects with the sub-effects (multiply coefficient with sub-effect), a total factorial effect can be found. This value will determine if the factor has a significant effect on the outcome. For larger numbers, the factor can be considered extremely important and for smaller numbers, the factor can be considered less important. The sign of the number also has a direct correlation to the effect being positive or negative. A null outcome situation is when the outcome of your experiment is the same regardless of how the levels within your experiment were combined.
The default factors are named "A", "B", "C", and "D" and have respective high and low levels of 1 and -1. The name of the factors can be changed by simply clicking in the box and typing a new name. Additionally, the low and high levels for each factor can be modified in this menu.
No comments:
Post a Comment